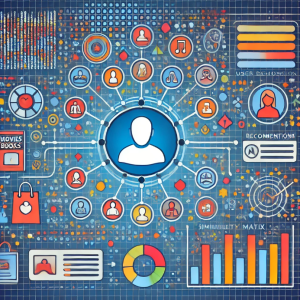
In this blog, we will learn about how to implement content-based recommender system using Python programming example. We will learn with the example of movie recommender system for recommending movies. Download the movies data from here to work with example given in this blog.
The following is a list of key activities we would do to build a movie recommender system based on content-based recommendation technique.
- Data loading & preparation
- Text vectorization
- Cosine similarity computation
- Getting recommendations
Data Loading & Preparation
To start with, we import the data in csv format. Once data is imported, next step is analyse and prepare data before we apply modeling techniques.
import pandas as pd
df = pd.read_csv('sample_data/movies.csv')
df = df[['title', 'genres', 'keywords', 'cast', 'director']]
df = df.fillna('') # Fill missing values with empty strings
df.head()
The dataset contains 24 columns, only a few of which are needed to describe a movie. In the above code, key features such as title, genres, etc are extracted and missing values in these features are filled with empty string.
Text Vectorization
The next step is add a column in the dataframe that holds the value created by combining other columns representing key attributes such as title, genres, etc. And, then CountVectorizer is used on that column to vectorize the text. The following code does the same.
from sklearn.feature_extraction.text import CountVectorizer
vectorizer = CountVectorizer(stop_words='english', min_df=20)
word_matrix = vectorizer.fit_transform(df['features'])
word_matrix.shape
The following happens in the above code:
- When instantiating Count_Vectorizer, stop_words = ‘english’, min_df = 20 is passed. The parameter, stop_words removes common English stop words (like “the”, “and”, “is”) that typically do not carry much meaning for analysis purposes. min_df=20 sets the minimum document frequency, meaning that only words that appear in at least 20 different documents will be included in the final matrix. This helps to filter out rare words that might add noise to the model.
- Next step is apply fit_transform method on df[‘features’] column. The fit part goes through the text data and learns the unique words (vocabulary), and builds a dictionary where each unique word is assigned an index. Once index is assigned, the next step is transform which is used to convert the text data into a numerical matrix, where each row corresponds to a document (or row in df), and each column represents a word (from the learned vocabulary). The matrix contains the counts of each word in the corresponding document.
- word_matrix.shape returns the dimension of the matrix, which is a sparse matrix. The shape represents (number of documents, number of words in vocabulary).
Cosine Similarity Computation
The table of word counts contains 4,803 rows—one for each movie—and 918 columns. The next task is to compute cosine similarities for each row pair:
from sklearn.metrics.pairwise import cosine_similarity
sim = cosine_similarity(word_matrix)
Getting Recommendation
The final step is to get one or more recommendations based on the input – a movie title. The Python code given below can be used to get the top N recommendations for movies similar to input movie name.
def recommend(title, df, sim, count=10):
# Get the row index of the specified title in the DataFrame
index = df.index[df['title'].str.lower() == title.lower()]
# Return an empty list if there is no entry for the specified title
if (len(index) == 0):
return []
# Get the corresponding row in the similarity matrix
similarities = list(enumerate(sim[index[0]]))
# Sort the similarity scores in that row in descending order
recommendations = sorted(similarities, key=lambda x: x[1], reverse=True)
# Get the top n recommendations, ignoring the first entry in the list since
# it corresponds to the title itself (and thus has a similarity of 1.0)
top_recs = recommendations[1:count + 1]
# Generate a list of titles from the indexes in top_recs
titles = []
for i in range(len(top_recs)):
title = df.iloc[top_recs[i][0]]['title']
titles.append(title)
return titles
The following can be learned from the above code:
- Similarity Matrices: The code demonstrates how to use a similarity matrix to recommend items based on their similarity to a given item. This is fundamental in content-based filtering, where recommendations are based on item attributes rather than user interactions.
- Ranking Similar Items: Sorting items based on similarity scores is a crucial step in ranking and recommending the most relevant items.
- Indexing and Filtering: The code uses df.index[df[‘title’].str.lower() == title.lower()] to filter and find the row index of the specified title. Understanding how to filter data in a DataFrame is a core skill when working with structured data.
- Lambda Functions: A lambda function is used to specify that sorting should be based on the second element of each tuple (the similarity score). This demonstrates how to create short, anonymous functions for specific tasks.
- Accessing Data by Index: The code retrieves titles using df.iloc[], demonstrating how to access rows and columns by index in a pandas DataFrame.
You can now invoke the recommend function to get the top 10 recommendations (count = 10). This is how you would invoke the function: recommend( ‘Spectre’, df, sim).
You can use the Python code example given in this blog to create a content-based recommender system. In case you have questions, feelf ree to drop a message.
- What is Embodied AI? Explained with Examples - May 11, 2025
- Retrieval Augmented Generation (RAG) & LLM: Examples - February 15, 2025
- How to Setup MEAN App with LangChain.js - February 9, 2025
I found it very helpful. However the differences are not too understandable for me