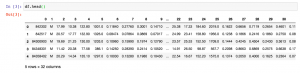
In this post, you will learn about when to use LabelEncoder. As a data scientist, you must have a clear understanding on when to use LabelEncoder and when to use other encoders such as One-hot Encoder. Using appropriate type of encoders is key part of data preprocessing in machine learning model building lifecycle.
Here are some of the scenarios when you could use LabelEncoder without having impact on model.
- Use LabelEncoder when there are only two possible values of a categorical features. For example, features having value such as yes or no. Or, maybe, gender feature when there are only two possible values including male or female.
- Use LabelEncoder for label columns in case of supervised learning when it is binary classification problem.
- Don’t use LabelEncoder when the categorical features have more than two values. The nominal categorical features having more than two values may get treated as ordinal one by the machine learning model. Although model performance won’t suffer much, it is recommended to use One-Hot encoder for categorical features having more than two different types of value. In such cases, one may want to use One-hot encoder, also called as dummy encoding. One can also use get_dummies method on Pandas package.
LabelEncoder Python Example
Here is the Python code which transforms the label binary classes into encoding 0 and 1 using LabelEncoder. The Breast Cancer Wisconsin dataset is used for illustration purpose. The information about this dataset can be found at https://archive.ics.uci.edu/ml/datasets/Breast+Cancer+Wisconsin+(Diagnostic). Note that LabelEncoder is class of sklearn.preprocessing package.
import pandas as pd
from sklearn.preprocessing import LabelEncoder
df = pd.read_csv(
'https://archive.ics.uci.edu/ml/'
'machine-learning-databases'
'/breast-cancer-wisconsin/wdbc.data',
header=None)
#
# Load the training data (X) and labels (y)
#
X = df.loc[:, 2:].values
y = df.loc[:, 1].values
#
# Instantiate LabelEncoder
#
le = LabelEncoder()
y = le.fit_transform(y)
Here is the how the data looks like:
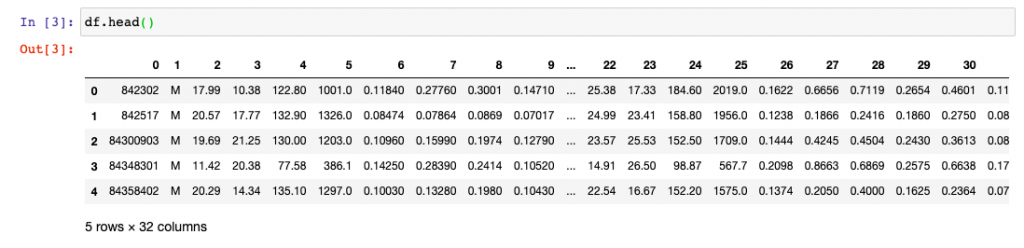
In case, you want to look at what all classes got transformed. You can use the following code representing attribute, classes_ on instance of LabelEncoder.
le.classes_
Read one related post on LabelEncoder – LabelEncoder example – single & multiple columns
- Retrieval Augmented Generation (RAG) & LLM: Examples - February 15, 2025
- How to Setup MEAN App with LangChain.js - February 9, 2025
- Build AI Chatbots for SAAS Using LLMs, RAG, Multi-Agent Frameworks - February 8, 2025
I found it very helpful. However the differences are not too understandable for me