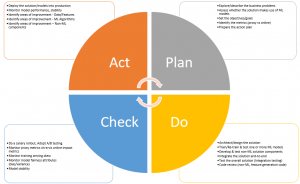
The primary goal of establishing and implementing Quality Assurance (QA) practices for machine learning/data science projects or, projects using machine learning models is to achieve consistent and sustained improvements in business processes making use of underlying ML predictions. This is where the idea of PDCA cycle (Plan-Do-Check-Act) is applied to establish a repeatable process ensuring that high-quality machine learning (ML) based solutions are served to the clients in a consistent and sustained manner.
The following diagram represents the details.
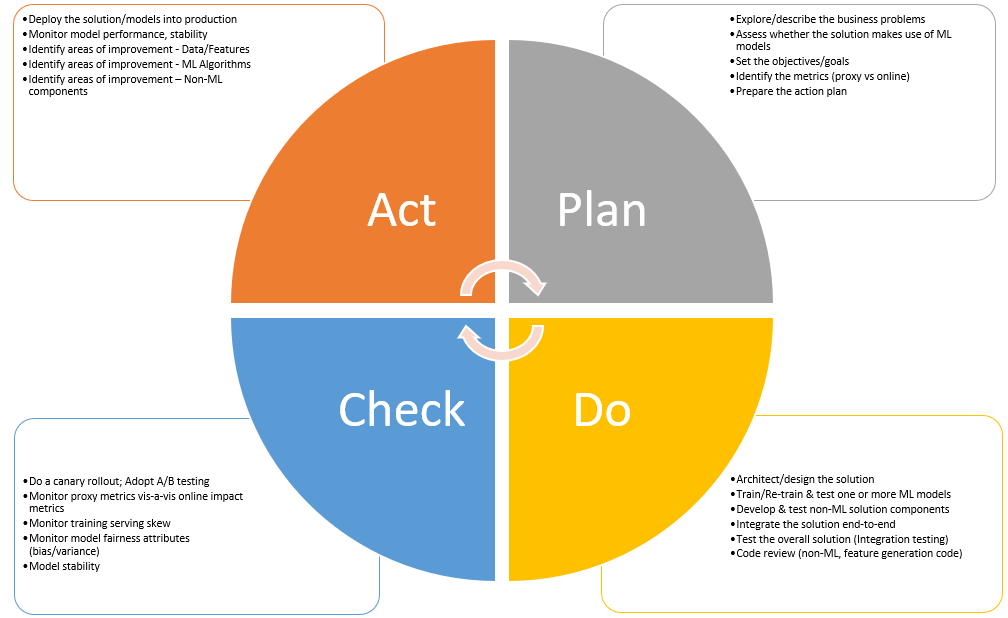
Fig1. PDCA cycle of machine learning projects for QA
The following represents the details listed in the above diagram.
-
Plan
-
Explore/describe the business problems: In this stage, product managers/business analyst sit with data scientist and discuss the business problem at hand. The outcome of this exercise is a product requirement specification (PRS) document which gets handed over to the data science team.
-
Assess whether the solution makes use of ML models: Data science team explores whether the solution needs one or more machine learning models to be built.
-
Set the objectives/goals: Product managers set the overall business objectives and goals to be met/realized as a result of ML solution being carved out.
-
Identify the metrics (proxy vs online): Suppose you want to measure how happy are users after visiting a specific web page of the website, the proxy metric could be the time spent by users on that page.
-
Prepare the action plan
-
-
Do (Implement)
-
Architect/design the solution
-
Train/Re-train & test one or more ML models: As part of building models, the following needs to be considered especially when improving on the previous models deployed into production:
-
Feature engineering to filter existing features and select new features to improve model performance
-
Update newer ML algorithms
-
-
Develop & test non-ML solution components
-
Integrate the solution end-to-end
-
Test the overall solution (Integration testing)
-
Code review (non-ML, feature generation code)
-
-
Check (Test/Monitor)
-
Do a Canary rollout; Adopt A/B testing
-
Monitor proxy metrics vis-a-vis online impact metrics
-
Monitor training serving skew
-
Monitor model fairness attributes (bias/variance)
-
Model stability (numerical stability)
-
-
Act (Standardize/Continuous Improvement)
-
Deploy the solution/models into production
-
Monitor model overall performance, stability, fairness (bias/variance)
-
Identify areas of improvement – Data/Features
-
Identify areas of improvement – ML Algorithms
-
Identify areas of improvement – Non-ML components
-
Reference
Summary
In this post, you learned about applying the PDCA cycle for managing the quality of machine learning (ML) / Data Science projects. The idea is to set up QA practices for ML projects. In the next posts, we will dive deeper into different aspects of Quality assurance practices.
- Retrieval Augmented Generation (RAG) & LLM: Examples - February 15, 2025
- How to Setup MEAN App with LangChain.js - February 9, 2025
- Build AI Chatbots for SAAS Using LLMs, RAG, Multi-Agent Frameworks - February 8, 2025
I found it very helpful. However the differences are not too understandable for me