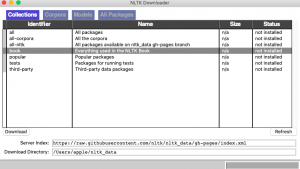
In this post, you will learn about getting started with natural language processing (NLP) with NLTK (Natural Language Toolkit), a platform to work with human languages using Python language. The post is titled hello world because it helps you get started with NLTK while also learning some important aspects of processing language. In this post, the following will be covered:
- Install / Set up NLTK
- Common NLTK commands for language processing operations
Install / Set up NLTK
This is what you need to do set up NLTK.
- Make sure you have Python latest version set up as NLTK requires Python version 3.5, 3.6, 3.7, or 3.8 to be set up. In Jupyter notebook, you could execute command such as !python –version to know the version.
- If you have got Anaconda set up, you can get started with executing command such as import nltk
- In case you don’t have Anaconda set up, you can execute the following command and get started. The command works well with Unix / Mac.
# Pip install
#
pip install nltk
#
# Import NLTK
#
import nltk
You could get started with practicing NLTK commands by downloading the book collection comprising of several books. Here is what you need to execute:
#
# NLTK Book Download
#
nltk.download()
Executing above command will open up a utility where you could select book and download. Here is how it looks like:
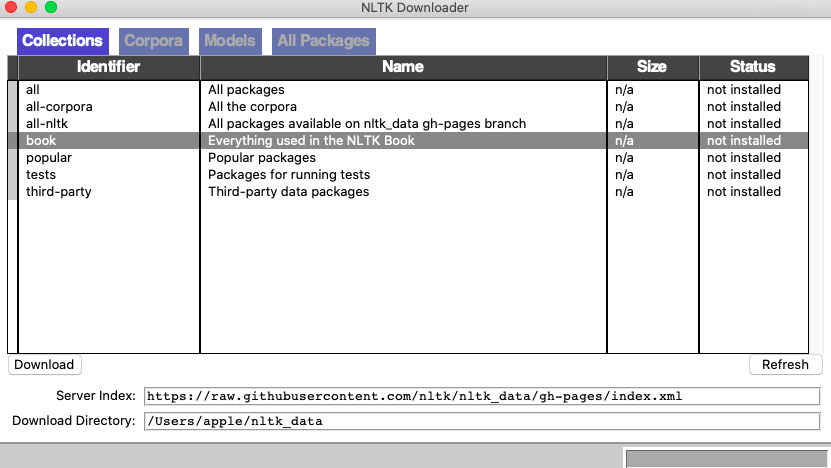
Select the book and click download. Once the download is complete, you could execute the following command to load the book.
#
# Load the books
#
from nltk.book import *
This is how it would look like by executing the above command.
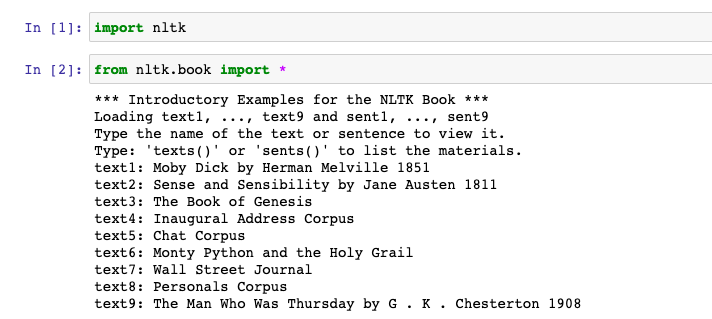
Common NLTK Commands / Methods for Language Processing
Here are some of the common NLTK commands vis-a-vis their utility:
- nltk.word_tokenize(): Tokenize the sentence in words and punctuations. This includes the duplicate words and punctuations as well.
import nltk
#
# Sentence
#
intro = 'My name is Ajitesh Shukla. I work in HighRadius. I live in Hyderabad.'
#
# Tokenize using word_tokenize method
#
tokens = nltk.word_tokenize(intro)
#
#
print(tokens)
#
#
print(set(tokens))
Here is how the output would look like:
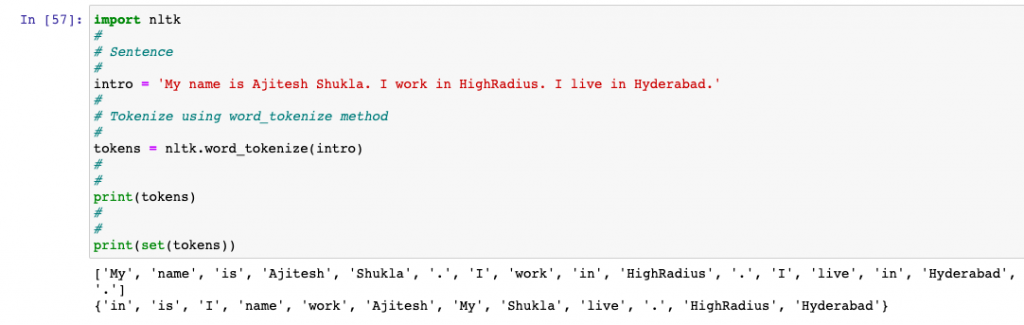
- concordance() vs similar() vs common_contexts(): These are three methods which could be invoked on nltk.text.Text objects in order to find the following:
- concordance: Find the context in which a word / token occurred. The output is a set of different sentences where the word occurred.
- similar: Find the similar context in which the word occurred. Basically, find the sentences that consist same surrounding words (left and right).
- common_contexts: Find the pair of common surrounding words of the tokens passed to common_contexts method.
We will try and understand with one of the text (text7 – Wall Street Journal) loaded from nltk.book. In the example below, common_contexts output is to_the and to_their. This implies that to_the and to_their occurred around both the words, finance and improve. If the output of common_contexts would have been null / empty, the output of method similar would also have been null / empty.
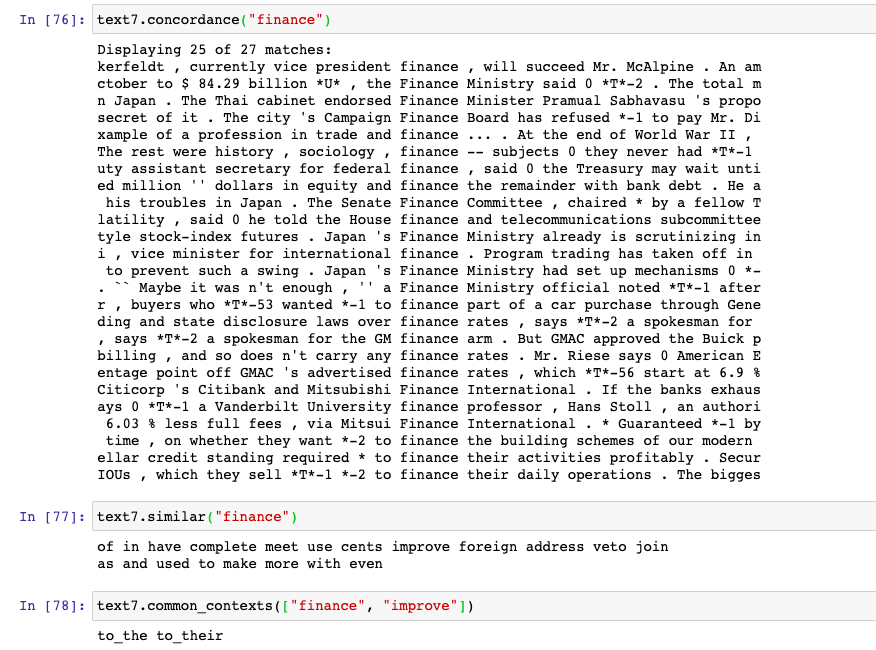
- FreqDist(): FreqDist() method counts the frequence of occurence of tokens (words or punctuations) and returns a FreqDist object representing a JSON object having key-value pair of key and number of occurrences. This method is present as part of nltk.probability package. You can use plot method on the instance of FreqDist to draw the plot.
import nltk
#
# Sentence
#
intro = 'My name is Ajitesh Shukla. I write blogs on Vitalflux.com. I live in Hyderabad. I love writing blogs. I also have good expertise in cloud computing. I am also good in AWS.'
#
# Tokenize using word_tokenize method
#
tokens = nltk.word_tokenize(intro)
#
# Create an instance of FreqDist
#
freqdist = FreqDist(tokens)
#
# Draw the frequency distribution of tokens
#
freqdist.plot()
Here is how the output plot would look like:
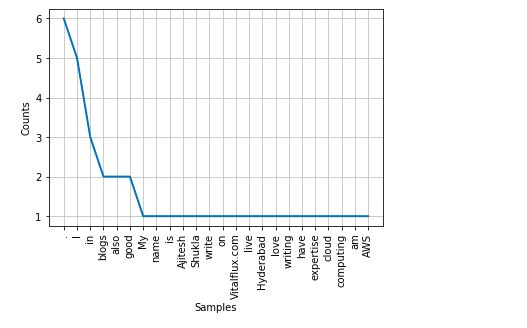
- Printing tokens (words) satisfying some property such as token length: Here is a piece of code which can be used to print all words which meet certain criteria. For example, length of word > 5. Note the last statement in the code given below:
import nltk
#
# Sentence
#
intro = 'My name is Ajitesh Shukla. I write blogs on Vitalflux.com. I live in Hyderabad. I love writing blogs. I also have good expertise in cloud computing. I am also good in AWS.'
#
# Tokenize using word_tokenize method
#
tokens = nltk.word_tokenize(intro)
#
# Condition to filter words meeting criteria
#
long_words = [words for words in tokens if len(words) > 5]
This is what will be printed
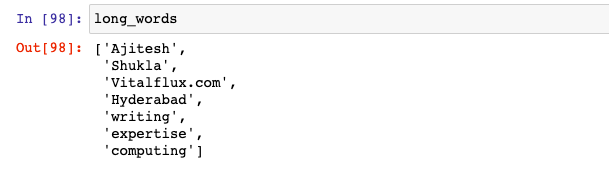
Conclusions
Here is the sumary of what you learned in this post related to NLTK set up and some common methods:
- NLTK is a Python library to work with human languages such as English.
- NLTK provides several packages used for tokenizing, plots etc.
- Several useful methods such as concordance, similar, common_contexts can be used to find words having context, similar contexts.
- Questions to Ask When Thinking Like a Product Leader - July 3, 2025
- Three Approaches to Creating AI Agents: Code Examples - June 27, 2025
- What is Embodied AI? Explained with Examples - May 11, 2025
I found it very helpful. However the differences are not too understandable for me