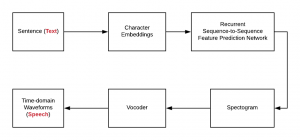
In this post, you would learn about a neural network reference solution architecture which could be used to convert the text to speech. The neural network solution architecture given in this post is based on deep learning (autoencoder network (encoder-decoder) with attention).
Neural Network Reference Architecture for Text-to-Speech Synthesis
In the solution architecture diagram (figure 1) depicted below, the following is described:
- Sentences are first converted into character embeddings. Character embeddings are numeric representations of words. Numeric representations of each of the words could be used to create numeric representations of higher-level representations like sentences/paragraphs/documents/etc.
- Character embeddings are next fed into recurrent sequence-to-sequence feature prediction network with attention. The sequence-to-sequence network predicts a sequence of the spectrogram. The feature prediction network consists of an encoder and a decoder network along with an attention network for converting / mapping character embeddings to a spectrogram.
- Encoder network: The encoder network encodes the character embeddings into a hidden feature representation.
- Attention network: The output of the encoder network can be consumed by an attention network which produces a fixed-length context vector for each decoder output step.
- Decoder network: The decoder network (autoregressive recurrent neural network – RNN) consumes output from the attention network and predicts the sequence of the spectrogram from the hidden feature representation.
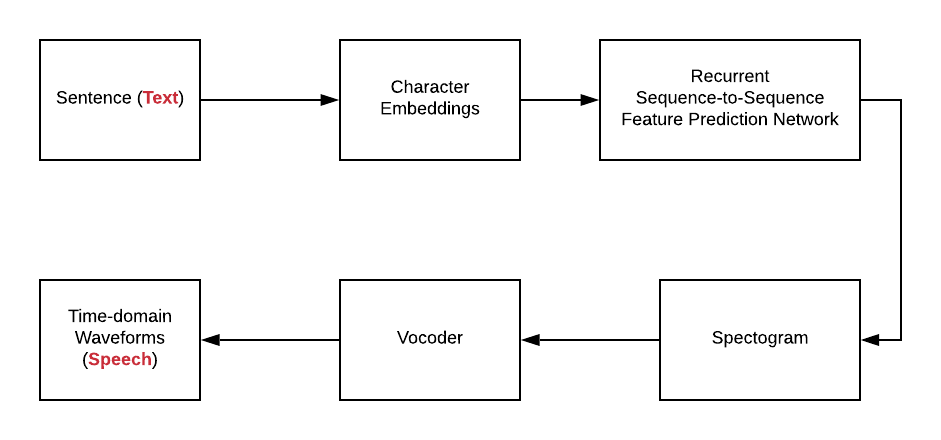
Fig 1. Neural Network Architecture for TTS
- The spectrogram is then fed into Vocoder which creates time-domain waveforms (Speech). The vocoder is short for Voice + Encoder. The vocoder is used to analyze and synthesize the human voice signal from the spectrogram. Wavenet is a classic example of a Vocoder. Wavenet is a generative model (deep neural network) of time-domain waveforms. It produces the human-like audio signal.
Reference
- Natural TTS synthesis by conditioning Wavenet on Mel Spectrogram predictions
- Vocoder
- Wavenet – A generative model for raw audio waveforms (signal)
- How does attention work in encoder-decoder network?
- Intuitive understanding of attention mechanism in deep learning
Summary
One of the emerging areas of AI / machine learning is the ability to clearly convert text to speech. In this field, deep learning has been extensively used to come up with unique and effective solutions. One of the solution architecture, as discussed in this post, makes use of converting the text into character embeddings and passing the embeddings through sequence-to-sequence prediction network (encoder-attention-decoder deep neural networks). The network converts the character embeddings into the spectrogram which could be further passed through Wavenet like the deep neural network to convert into raw human-like audio (time-domain waveforms) signals.
- Retrieval Augmented Generation (RAG) & LLM: Examples - February 15, 2025
- How to Setup MEAN App with LangChain.js - February 9, 2025
- Build AI Chatbots for SAAS Using LLMs, RAG, Multi-Agent Frameworks - February 8, 2025
I found it very helpful. However the differences are not too understandable for me