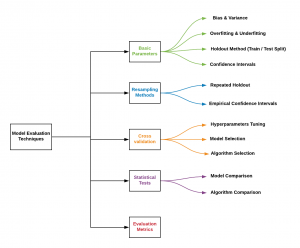
In this post, you will get an access to a self-explanatory infographics / diagram representing different aspects / techniques which need to be considered while doing machine learning model evaluation. Here is the infographics:
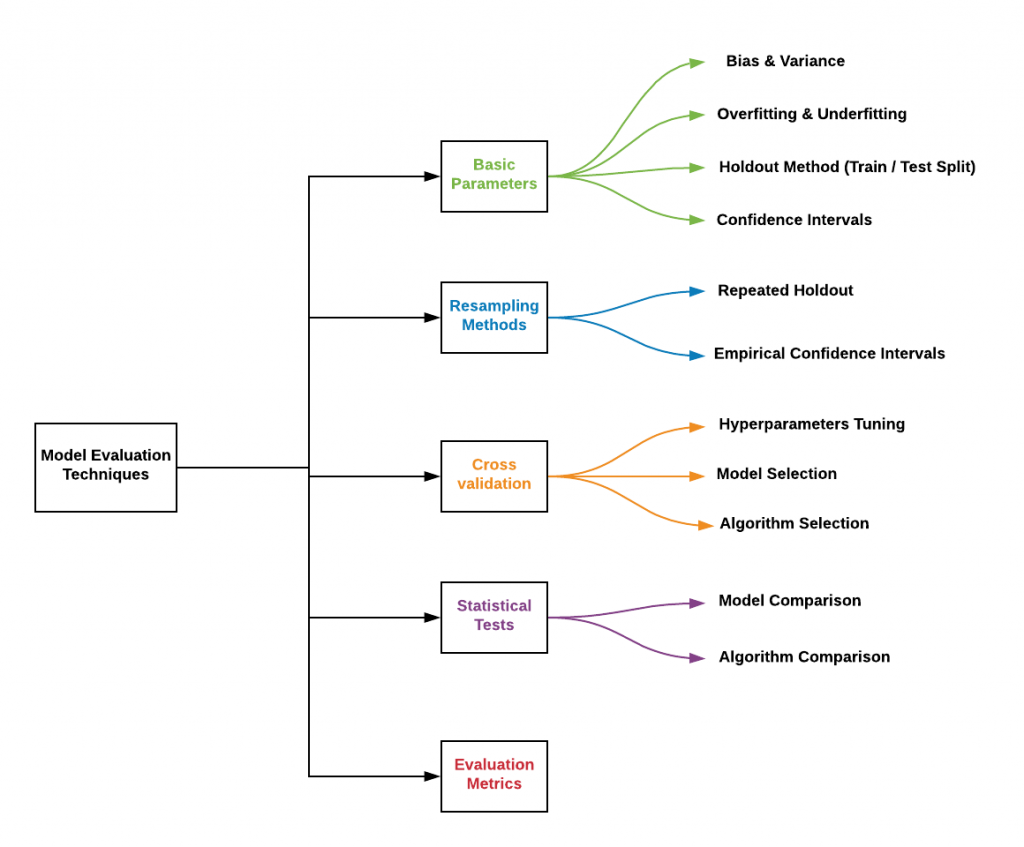
In the above diagram, you will notice that the following needs to be considered once the model is trained. This is required to be done to select one model out of many models which get trained.
- Basic parameters: The following need to be considered for evaluating the model:
- Bias & variance
- Overfitting & underfitting
- Holdout method
- Confidence intervals
- Resampling methods: The following techniques need to be adopted for evaluating models:
- Repeated holdout
- Empirical confidence intervals
- Cross-validation: Cross validation technique is required to be performed for achieving some of the following
- Hyperparameters tuning
- Model selection
- Algorithm selection
- Statistical tests: Statistical tests need to be performed for doing the following:
- Model comparison
- Algorithm comparison
- Evaluation metrics
The image is adopted from this page.
Latest posts by Ajitesh Kumar (see all)
- What are AI Agents? How do they work? - January 7, 2025
- Agentic AI Design Patterns Examples - January 6, 2025
- List of Agentic AI Resources, Papers, Courses - January 5, 2025
I found it very helpful. However the differences are not too understandable for me