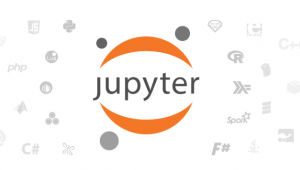
Are you starting to create machine learning models (using python programming) using JupyterLab or Jupyter Notebook? This post list down some commands which are found to be very useful while one (beginner data scientist) is getting started with using JupyterLab notebook for building machine learning models.
- Notebook Operations: The following command helps to perform operations with the notebook.
- Ctrl + S: Save the notebook
- Ctrl + Q: Close the notebook
- Enter: While on any cell, you want to enter edit mode, press Enter.
- Cells Operation: The following commands help with performing operations on cells:
- J: Select the cell below the current cell; This command would be used to go through cells below the current cell; Once you reached to the desired cell, you could Shift + Enter to execute the command in the given cell.
- K: Select the cell above the current cell
- X: Cut the content of the current cell
- C: Copy the content of the current cell
- V: Paste the command copied into the below cell
- Shift + Enter: Run the selected cell and insert a cell below
- Ctrl + Enter: Run the selected cell but do not add the cell below; If this command is used, one could use B to enter a cell below.
- B: Enter a cell below. This command comes handy for entering the cell at any point. Very useful if you want to add a markdown note before/after execution of any command.
- D,D: Delete the cell
- Notes taking operations using Markdown editor: If you are a blogger, you would love these shortcuts. Very useful for taking notes while you are working on your models.
- M: Go to Markdown mode to write some notes in markdown format
- Y: Go to Code mode to continue working with code
Summary
In this post, you learned about some of the quick commands (Cheat sheet) which you could use for your reference while working with JupyterLab or Jupyter Notebook. Please feel free to suggest other commands that you found to be useful which are not listed above.
- What are AI Agents? How do they work? - January 7, 2025
- Agentic AI Design Patterns Examples - January 6, 2025
- List of Agentic AI Resources, Papers, Courses - January 5, 2025
I found it very helpful. However the differences are not too understandable for me