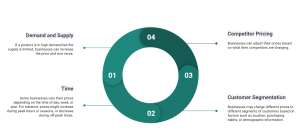
Are you a product manager looking to maximize profits and improve product performance, or a data scientist eager to leverage the power of machine learning to solve complex business problems related to dynamic pricing? Do you ever wonder how businesses can optimize their pricing strategies to stay competitive, cater to customer expectations, and enhance their market positioning?
In this blog, we uncover the intersection of advanced AI technologies with smart pricing strategies. In an era where customer expectations, market trends, and competitor actions change rapidly, businesses need an agile and data-driven approach to pricing. That’s where dynamic pricing coupled with machine learning comes into play. We’ll explore compelling strategies, reveal the key business drivers behind them, and illustrate with real-world examples. This blog aims to provide valuable insights for both product managers who wish to understand and implement effective pricing strategies, and data scientists looking to broaden their knowledge about practical applications of machine learning in business optimization.
What is Dynamic Pricing?
Dynamic pricing, also known as demand-based pricing or time-based pricing, is a pricing strategy in which businesses set flexible prices for products or services based on current market demands. Rather than setting a static price, businesses adjust prices in response to various factors such as:
- Demand and Supply: If a product is in high demand but the supply is limited, businesses can increase the price. Conversely, if a product is not in high demand or if there is a surplus, businesses can reduce the price to boost sales.
- Competitor Pricing: Businesses can adjust their prices based on what their competitors are charging.
- Customer Segmentation: Businesses may charge different prices to different segments of customers based on factors such as location, purchasing habits, or demographic information.
- Time: Some businesses vary their prices depending on the time of day, week, or year. For instance, prices might increase during peak hours or seasons, or decrease during off-peak times.
Dynamic pricing allows businesses to maximize their profits by capitalizing on market trends and customer behavior. It helps match prices to a customer’s willingness to pay, which can lead to increased sales. It also allows businesses to quickly respond to changes in the market, keeping them competitive.
AI and machine learning can be powerful tools for implementing dynamic pricing strategies. They can analyze vast amounts of data in real time, predict trends, and make recommendations for price adjustments.
For instance, machine learning algorithms can use historical sales data, competitor pricing information, and customer behavior data to predict how changes in price might affect demand. They can then use this information to recommend optimal prices. Similarly, AI can be used to segment customers based on their behavior and preferences, enabling businesses to set prices that maximize revenue for each segment.
In addition, AI and machine learning can be used to automate the dynamic pricing process. Instead of manually analyzing data and adjusting prices, businesses can use AI to automatically adjust prices in response to changes in the market.
Dynamic Pricing Use Cases & Machine Learning
Dynamic pricing leverages machine learning in various ways to optimize pricing strategies. Here are some specific use cases along with business drivers, data requirements and real-life example.
- Demand Forecasting: Machine learning can be used to predict future demand for a product based on historical data and other relevant factors like seasonality, promotions, and market trends. These predictions can help businesses adjust their prices to maximize revenue or profit.
- Business Driver: Maximizing sales and profits, optimizing inventory, and improving customer satisfaction by ensuring products or services are available when customers want them.
- Data needed: Historical sales data, pricing data, promotional activity data, external factors data such as holidays, seasonality, or market trends.
- Real-life Example: Retailers like Amazon and Walmart use demand forecasting to adjust their prices in real-time, balancing their supply and demand while maximizing profit.
- Competitive Pricing Analysis: Machine learning algorithms can analyze competitors’ prices and use this information to make strategic pricing decisions. For instance, a business might want to set its prices slightly lower than its competitors to attract price-sensitive customers.
- Business Driver: Staying competitive in the market, attracting price-sensitive customers, and protecting market share.
- Data Needed: Competitor pricing data, own pricing and sales data, product feature data.
- Real-life Example: Online travel agencies like Expedia or Booking.com adjust their hotel rates based on competitive pricing analysis to attract customers who are comparing prices on different platforms.
- Personalized Pricing: By analyzing customer data, machine learning can segment customers based on their purchasing behavior, demographics, or preferences. These insights can be used to offer personalized prices, potentially increasing conversion rates and customer satisfaction.
- Business Driver: Increasing conversion rates, improving customer satisfaction, and driving customer loyalty by offering personalized deals.
- Data Needed: Customer behavior data, demographic data, purchase history data, browsing history data.
- Real-life Example: E-commerce platforms like Alibaba or JD.com leverage personalized pricing to offer discounts to specific customer segments, thereby increasing conversion rates and enhancing customer satisfaction.
- Price Optimization: Machine learning can be used to find the optimal price for a product that maximizes revenue or profit. This often involves exploring different price levels, predicting how these will affect sales, and choosing the price that yields the highest revenue or profit.
- Business Driver: Maximizing revenue and profit by setting the most profitable price.
- Data Needed: Historical sales data, pricing data, cost data, demand elasticity data.
- Real-life Example: Companies like PROS or Pricefx offer price optimization solutions that help businesses like airlines, manufacturers, and distributors set optimal prices for their products or services.
- Dynamic Pricing Automation: Machine learning can automate the dynamic pricing process, continuously analyzing market data and adjusting prices in real time. This can save a significant amount of time and resources compared to manual price adjustments.
- Business Driver: Reducing the manual workload, making real-time pricing decisions, and responding rapidly to market changes.
- Data Needed: Real-time market data, historical sales data, pricing data, competitive pricing data.
- Real-life Example: Uber uses dynamic pricing automation to adjust ride fares in real-time based on current demand and supply in different locations.
- Surge Pricing: In industries like ride-sharing or hotel booking, machine learning can be used to implement surge pricing, where prices are increased during periods of high demand.
- Business Driver: Balancing demand and supply in real-time, preventing service disruptions due to high demand, and maximizing revenue during peak times.
- Data Needed: Real-time demand and supply data, location data, time data.
- Real-life Example: Uber and Lyft use surge pricing during peak times, such as rush hour or during a large event, when the demand for rides is higher.
- Inventory Management: By predicting demand and adjusting prices accordingly, machine learning can also help businesses manage their inventory more efficiently, reducing holding costs and the risk of stockouts or overstocking.
- Business Driver: Reducing inventory costs, preventing stock outs or overstocking, and improving operational efficiency.
- Data Needed: Inventory level data, historical sales data, demand forecasting data.
- Real-life Example: Retailers like Amazon use machine learning for inventory management, adjusting prices based on current stock levels and predicted future demand.
- Yield Management: Particularly relevant in the airline and hotel industry, machine learning can be used to adjust prices dynamically based on factors like booking lead time, occupancy rates, and cancellations, with the goal of maximizing revenue from a fixed, perishable inventory (such as airline seats or hotel room nights).
- Business Driver: Maximizing revenue from a fixed, perishable inventory, improving resource utilization, and optimizing demand during different periods.
- Data Needed: Booking data, cancellation data, inventory level data, demand forecasting data, and historical pricing data.
- Real-life Example: Airlines like Delta and hotels like Marriott use yield management to adjust prices dynamically. For example, as the date of a flight or hotel stay gets closer, prices may increase based on predicted demand and remaining inventory.
Conclusion
Dynamic pricing, powered by machine learning has become an essential business strategy for several organizations. From forecasting demand to optimizing prices, the convergence of these two domains offers endless possibilities for businesses to stay ahead of the curve, maximize profits, and meet customer expectations.
As we’ve seen through the different strategies and real-world examples, implementing dynamic pricing is not just about making real-time adjustments to prices. It also involves understanding your customers, tracking market trends, and analyzing competitor pricing models. The intelligent use of machine learning algorithms can provide the critical insights needed for these tasks, offering a competitive edge in today’s fast-paced, data-driven world. For product managers, the key takeaway is the importance of a flexible and data-driven approach to pricing, which can significantly enhance product performance and profitability. For data scientists, the intersection of machine learning and dynamic pricing presents a compelling field of study and a myriad of opportunities to apply advanced algorithms to solve complex business problems.
- Retrieval Augmented Generation (RAG) & LLM: Examples - February 15, 2025
- How to Setup MEAN App with LangChain.js - February 9, 2025
- Build AI Chatbots for SAAS Using LLMs, RAG, Multi-Agent Frameworks - February 8, 2025
I found it very helpful. However the differences are not too understandable for me