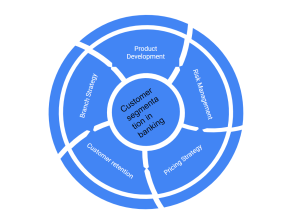
Ever wondered how some banks seem to know exactly what their customers need, almost before the customers do? They’re probably leveraging the power of customer segmentation. We all know how vital it is for any businesses including banks to truly understand their customers in today’s competitive landscape. And that’s where the magic of customer segmentation comes into play. It is enabling banks to dive deep into customer data and extract actionable insights, influencing everything from crafting personalized experiences to strategic decision making.
In this blog post, we’re going to learn about customer segmentation use cases in banking, showcasing how it’s going to impact product development, risk management, and customer acquisition. Machine learning is taking customer segmentation from ‘helpful’ to ‘indispensable,’ opening up new avenues and making the whole process smarter and more efficient. Whether you’re a product manager wanting to fine-tune your segmentation strategy or a data scientist curious about how machine learning is revolutionizing the banking industry, we will learn about key concepts related to customer segmentation.
Customer Segmentation Use Cases in Banking
Customer segmentation is an incredibly versatile tool and can aid in various business applications in the banking industry beyond personalizing the omnichannel experience. Machine learning algorithms can analyze vast and diverse datasets to identify patterns that may not be apparent to human analysts. This could include transactional data, demographic information, web browsing behavior, social media activity, customer service interactions, and more. By effectively utilizing ML, banks can understand their customers in much more nuanced ways and form more precise segments. The following represents some key use cases of customer segmentation in banking:
Here are a few examples of how customer segmentation can leverage machine learning for different use cases in banking:
- Product Development: Understanding the needs and preferences of different customer segments can inform the development of new banking products and services. For instance, a segment of environmentally-conscious customers might be interested in ‘green’ investment products. Machine learning can help identify this customer segment by analyzing various data points such as the following. Once the bank has identified an environmentally-conscious customer segment using ML models trained on some of the following data points, they can tailor their product development efforts accordingly. This could involve developing green investment products, such as ESG (Environmental, Social, and Governance) funds, green bonds, or sustainable ETFs. The following data can be used for training the model:
- Transactional Data: ML algorithms can analyze customers’ transaction histories to identify those who frequently shop at eco-friendly stores or buy sustainable products.
- Demographics and Surveys: Algorithms can analyze demographic data and survey responses to identify customers who are likely to be environmentally conscious. For instance, research shows that younger generations are often more concerned about environmental issues.
- Social Media Activity: Machine learning can also analyze social media data to identify customers who frequently engage with environmental content or follow green organizations.
- Web Browsing Behavior: By analyzing cookies and web browsing data, ML can identify customers who frequently visit environmentally-focused websites or search for information about green investments.
- Risk Management: Different customer segments may have different risk profiles. For example, young customers with irregular income might be considered higher risk compared to older customers with stable income. Segmentation can help banks better understand and manage these risks. The following represents steps that can be used for training machine learning models that predict customer in different segments related to different risk profiles.
- Identifying Risk Factors: The first step is to identify what factors contribute to risk. These might include age, income stability, employment status, credit history, existing debts, and many others. These features form the basis for training ML models. Banks typically have access to this kind of data from account application forms, credit bureau reports, and transaction history.
- Building Predictive Models: Using the identified features, banks can train ML models to predict risk levels for individual customers. These models could use techniques like logistic regression, decision trees, or neural networks, depending on the complexity of the data. For instance, if ‘young customers with irregular income’ is identified as a high-risk segment, the ML model can be trained to assign higher risk scores to customers in this segment.
- Continuous Learning: One of the significant advantages of ML models is their ability to learn and improve over time. As the models are exposed to more data (e.g., new customers, instances of defaults or delinquencies), they can refine their predictions to become more accurate.
- Risk Mitigation Strategies: Once the models are in place, banks can develop risk mitigation strategies based on the ML predictions. For high-risk segments, this could involve requiring additional collateral, charging higher interest rates, or offering financial education services.
- Personalized Risk Management: Banks can also personalize their risk management strategies using the insights derived from ML models. For example, for customers who are identified as high-risk due to irregular income, banks might offer products with flexible payment schedules to accommodate income volatility.
- Pricing Strategy: Banks can use customer segmentation to develop tiered pricing strategies. Customers with more assets or higher transaction volumes might receive more favorable rates.
- Customer Retention: By understanding why certain customer segments might leave (using churn analysis), banks can develop strategies to improve retention. For example, if customers in a certain segment frequently leave due to high fees, the bank could consider a revised fee structure.
- Cross-Selling and Up-Selling: Customer segmentation can inform cross-selling and up-selling strategies. For instance, a bank could identify a segment of customers who use their credit card frequently and offer them a premium card with additional benefits.
- Branch Strategy: Customer segmentation can guide decisions about where to locate new branches or ATMs, what services to offer at each branch, and when to transition services to digital channels. For example, if a particular geographic area has a high concentration of small business owners, a new branch there could focus on serving their needs.
- Customer Acquisition: By understanding which customer segments are most profitable or have the most growth potential, banks can target their acquisition strategies more effectively. For example, a bank might launch a marketing campaign aimed at attracting more customers in the lucrative ‘young professionals’ segment.
Conclusion
In an era where data is abundant and technology continues to advance at lightning speed, banks must leverage tools like machine learning and customer segmentation to stay competitive and customer-focused. From product development to risk management, these techniques provide a powerful means to understand and address diverse customer needs, tailor experiences, and drive overall business performance.
Banks that successfully employ ML-driven customer segmentation will have the distinct advantage of being able to anticipate customer needs, create personalized offerings, and build stronger, more profitable relationships. In this landscape, the question isn’t whether banks should adopt machine learning and customer segmentation – it’s how fast they can adapt and innovate using these tools.
- Retrieval Augmented Generation (RAG) & LLM: Examples - February 15, 2025
- How to Setup MEAN App with LangChain.js - February 9, 2025
- Build AI Chatbots for SAAS Using LLMs, RAG, Multi-Agent Frameworks - February 8, 2025
I found it very helpful. However the differences are not too understandable for me