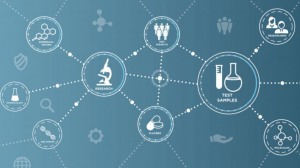
Are you a statistician, data scientist or business analyst working in the field of clinical trials? Do you find yourself curious about how statistical analyses play a pivotal role in unlocking valuable actionable insights and driving critical decisions in drug development? If so, in this blog, we will learn about various different use cases where clinical trials and statistics intersect.
Clinical trials are the backbone of evidence-based medicine, paving the way for the discovery and development of innovative therapies that can improve patient outcomes. Within this realm, statistics allows researchers and analysts to make sense of complex data, evaluate treatment efficacy, assess safety profiles, and optimize trial design. In this blog, we will delve into several real-world use cases where statistics takes center stage, empowering data scientists, statisticians and business analysts to unravel the mysteries hidden within clinical trial data. We will learn about the statistical tests employed for various use cases in clinical trials and, hence, drug development. We will explore how efficacy assessments of new drugs can be performed, safety evaluations can be conducted, optimal dosages can be determined, and comparative analyses can shed light on the relative effectiveness of different treatments.
Clinical Trials & Statistics Use Cases
The following represents some of the clinical trials use cases and how statistical tests play important role related to realizing the business goals of drug development:
Efficacy Assessment
Efficacy assessment, in the context of clinical trials, refers to the evaluation of a drug’s effectiveness or its ability to produce a desired therapeutic effect. It involves analyzing and interpreting data collected during the clinical trial to determine whether the experimental treatment or intervention demonstrates a significant and favorable outcome compared to a control group or alternative treatments.
The following are some of the statistical tests that can be used for efficacy assessment in clinical trials:
- T-Tests: T-tests are commonly employed to compare means between two groups, such as a treatment group and a control group. They can assess whether there is a significant difference in the outcome measures between the two groups, providing evidence of efficacy.
- Analysis of Variance (ANOVA): ANOVA is used when there are more than two groups involved in the comparison. It allows for the evaluation of treatment effects across multiple arms or different dosage levels. ANOVA helps determine whether there are statistically significant differences in outcomes among the groups.
- Survival Analysis: Survival analysis techniques, such as the Kaplan-Meier method and Cox proportional hazards models, are used when the primary endpoint is a time-to-event outcome, such as overall survival or progression-free survival. These analyses assess the impact of the treatment on survival rates, helping determine the drug’s efficacy in prolonging patient survival.
- Chi-Square Test: The chi-square test is often used when analyzing categorical data or evaluating the relationship between treatment groups and categorical outcomes. It can assess whether there are significant differences in response rates or treatment success rates between different arms of the trial.
- Non-Inferiority or Equivalence Testing: In some cases, the goal of a clinical trial may not be to demonstrate superiority but rather to establish non-inferiority or equivalence to a comparator treatment. Specialized statistical tests, such as non-inferiority testing, can be employed to evaluate whether the experimental treatment is not significantly worse than the comparator treatment by a predefined margin.
Safety Evaluation
Safety evaluation in clinical trials involves assessing the safety profile and potential adverse effects of an investigational drug. It ensures patient safety by identifying and evaluating risks and adverse events. It also aids in risk-benefit analysis, regulatory compliance, accurate adverse event reporting, risk management, and ongoing pharmacovigilance. Safety evaluation is critical for making informed decisions about patient welfare, determining the balance between benefits and risks, meeting regulatory requirements, mitigating risks, and monitoring long-term safety.
Safety evaluation involves monitoring and analyzing data collected during the trial to identify and evaluate any adverse events, side effects, or risks associated with the experimental treatment. The following are key focus areas related to safety evaluation and related questions / hypothesis and statistical tests:
The top three focus areas related to safety evaluation in clinical trials are:
- Adverse Events and Side Effects: a) What are the reported adverse events associated with the investigational drug?
- Hypothesis: The incidence of adverse events in the treatment group will be higher compared to the control group. b) What is the severity and frequency of the reported adverse events?
- Chi-Square Test to compare the incidence of adverse events between the treatment and control groups.
- Hypothesis: The severity of adverse events will be mild to moderate, and the frequency will be higher in the initial treatment period. c) Are there any specific patient characteristics that may influence the occurrence of adverse events?
- Descriptive statistics can be used to calculate the mean severity score and frequency distribution of adverse events.
- Hypothesis: Elderly patients will experience a higher incidence of adverse events compared to younger patients due to age-related factors.
- Logistic Regression can be used to analyze the association between patient characteristics (e.g., age, gender) and the occurrence of adverse events.
- Hypothesis: The incidence of adverse events in the treatment group will be higher compared to the control group. b) What is the severity and frequency of the reported adverse events?
- Laboratory and Clinical Measurements: a) Are there any significant changes in laboratory parameters or clinical measurements?
- Hypothesis: Treatment with the investigational drug will lead to improvements in disease-specific biomarkers compared to the control group. b) Are there any unexpected abnormalities or outliers in laboratory or clinical measurements?
- Paired t-tests or Wilcoxon signed-rank tests to compare pre- and post-treatment measurements within the treatment group.
- Data visualization techniques (e.g., box plots) to identify outliers and statistical tests (e.g., Grubbs’ test) to confirm their significance.
- Hypothesis: Certain laboratory parameters, such as liver function tests, may show transient abnormalities, but they will return to baseline levels without clinical significance. c) Do changes in laboratory or clinical measurements correlate with the occurrence of adverse events?
- Hypothesis: Patients experiencing certain adverse events, such as gastrointestinal symptoms, will show corresponding abnormalities in laboratory parameters related to gastrointestinal function.
- Hypothesis: Treatment with the investigational drug will lead to improvements in disease-specific biomarkers compared to the control group. b) Are there any unexpected abnormalities or outliers in laboratory or clinical measurements?
- Drug Interactions and Contraindications: a) Are there any potential drug-drug interactions with the investigational drug and concomitant medications?
- Hypothesis: The investigational drug will not have clinically significant interactions with commonly prescribed medications, based on preclinical and early-phase clinical trial data. b) Are there any contraindications or precautions identified for specific patient populations or coexisting medical conditions?
- Hypothesis: Patients with a history of cardiovascular disease will have a higher risk of adverse events related to cardiac function due to the investigational drug’s mechanism of action. c) Are there any unexpected effects observed when the investigational drug is administered in combination with other therapies?
- Hypothesis: Combining the investigational drug with a standard-of-care medication will result in improved efficacy without an increased risk of adverse events compared to the standard-of-care medication alone.
Dose Determination
Dose determination in clinical trials is the process of identifying the optimal dosage level, frequency, and regimen of an investigational drug to achieve the desired therapeutic effect while ensuring patient safety. It involves evaluating different doses to maximize efficacy and minimize adverse events. By finding the right dose, drug developers can tailor treatment to individual patient characteristics and enhance market positioning. Dose determination is crucial for regulatory approval, treatment optimization, and improving overall drug development strategies, ultimately leading to improved treatment outcomes and patient well-being.
The top three focus areas related to dose determination along with questions / hypothesis are following:
Optimal Dosage Level: Optimal dosage level is about determining the most effective dosage of the investigational drug to achieve the desired therapeutic effect. Here are few key questions & hypotheses.
- Question: What is the optimal dosage level of the investigational drug to achieve the desired therapeutic effect?
- Hypothesis: Increasing the dosage of the investigational drug will lead to a higher response rate or greater improvement in the primary endpoint.
- Statistical Test: Regression analysis or analysis of variance (ANOVA) to assess the relationship between dosage level and treatment response.
- Question: What is the relationship between dosage level and safety profile?
- Hypothesis: Higher dosages of the investigational drug will be associated with an increased incidence of adverse events or dose-limiting toxicities.
- Statistical Test: Chi-square test or logistic regression to compare the incidence of adverse events between different dosage groups.
- Question: Are there any specific patient characteristics that may influence the optimal dosage response?
- Hypothesis: Patients with a specific genetic polymorphism will require a lower dosage of the investigational drug to achieve the desired therapeutic effect.
- Statistical Test: Interaction analysis or subgroup analysis to evaluate the effect of the genetic polymorphism on treatment response at different dosage levels.
Dose Frequency and Regimen: Dose frequency and regimen is about investigating the most appropriate timing and frequency of administration for the investigational drug to optimize treatment outcomes and patient adherence. Here are few key questions & hypotheses.
- Question: What is the optimal frequency of administration for the investigational drug?
- Hypothesis: More frequent dosing of the investigational drug will result in better treatment outcomes compared to less frequent dosing.
- Statistical Test: Chi-square test or logistic regression to compare treatment outcomes between different dosing frequencies.
- Question: Is there a difference in efficacy or safety when the investigational drug is administered once daily versus multiple times daily?
- Hypothesis: Once-daily dosing of the investigational drug will be non-inferior to multiple times daily dosing in terms of efficacy and safety.
- Statistical Test: Non-inferiority testing or equivalence testing to compare treatment outcomes between once-daily and multiple times daily dosing regimens.
- Question: How does the dosing regimen of the investigational drug impact patient adherence and compliance?
- Hypothesis: Simplifying the dosing regimen (e.g., reducing the number of daily doses) will improve patient adherence and compliance.
- Statistical Test: Comparison of adherence rates or compliance scores between different dosing regimens using t-tests or chi-square tests.
Dose Individualization and Patient Factors: Dose individualization and patient factors is about tailoring the dosage of the investigational drug based on the consideration of patient-specific characteristics, such as age, weight, genetic factors, and coexisting medical conditions. Here are few key questions & hypotheses.
- Question: Can the dosage of the investigational drug be individualized based on patient-specific factors?
- Hypothesis: Patient weight will significantly impact the optimal dosage calculation, and individualized dosing will lead to better treatment outcomes.
- Statistical Test: Regression analysis or correlation analysis to evaluate the relationship between patient weight and optimal dosage calculation.
- Question: Are there any demographic or physiological factors that influence the pharmacokinetics and pharmacodynamics of the investigational drug?
- Hypothesis: Elderly patients will require lower dosages due to age-related changes in drug metabolism and clearance.
- Statistical Test: Analysis of covariance (ANCOVA) or regression analysis to assess the influence of age on dosage requirements while controlling for other factors.
- Question: How do coexisting medical conditions affect the recommended dosage of the investigational drug?
- Hypothesis: Patients with impaired renal function will require dosage adjustments to maintain efficacy and minimize the risk of drug accumulation.
- Statistical Test: Pharmacokinetic analysis or clearance estimation techniques to determine the appropriate dosage adjustments based on renal function measurements.
These hypotheses can guide the design and analysis of dose determination studies in clinical trials. By formulating these questions and hypotheses, researchers can plan statistical analyses to assess dose-response relationships, identify optimal dosage levels, evaluate safety profiles, and understand the impact of patient factors on dosing requirements.
- Completion Model vs Chat Model: Python Examples - June 30, 2024
- LLM Hosting Strategy, Options & Cost: Examples - June 30, 2024
- Application Architecture for LLM Applications: Examples - June 25, 2024
Leave a Reply