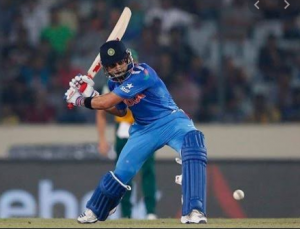
This post represents a real-world example of Binomial and Beta probability distribution from the sports field. In this post, you will learn about how the run scored by a Cricket player could be modeled using Binomial and Beta distribution. Ever wanted to predict the probability of Virat Kohli scoring a half-century in a particular match. This post will present a perspective on the same by using beta distribution to model the probability of runs that can be scored in a match. If you are a data scientist trying to understand beta and binomial distribution with a real-world example, this post will turn out to be helpful.
First and foremost, let’s identify the random variable that we would like to study. In this post, lets set the random variable as the half-century (runs equal to or more than 50) scored by a player in a match.
X = Half-century (runs equal to or more than 50) scored by a player in a match
First and foremost, let’s identify the random variable that we would like to study. In this post, lets set the random variable as the half-century (runs equal to or more than 50) scored by a player in a match. If the player scores a half-century, the random variable takes the value of SUCCESS (X = 1). If the player does not score a half-century, the random variable takes the value of failure (X = 0).
Representing Runs Scored as Binomial Distribution
Taking the last 30 matches in the consideration, there will be N number of successes (half-century scored) and (30-N) number of failures. This can be modeled using Binomial Distribution (a series of successes and failures).
Representing Probability of Runs as Beta Distribution
Let’s say we would like to predict the likelihood of whether the player will score a half-century in the upcoming cricket match. This problem could be modeled using Beta Distribution as the likelihood of the player scoring half-century could take value anywhere in the range of [0, 1]. Note the fact that we are predicting prior expectations.
Given our current problem, the best way to represent these prior expectations is with the Beta distribution. We are roughly expecting whether the player will score half-century even before the player has played the first ball of the match. Let’s say that it is expected that the probability that the player will score half-century will be most likely around .27, but that it could reasonably range from .21 to .35. The following plot will represent the beta distribution.
References
Intuition behind Beta Distribution
- What is Embodied AI? Explained with Examples - May 11, 2025
- Retrieval Augmented Generation (RAG) & LLM: Examples - February 15, 2025
- How to Setup MEAN App with LangChain.js - February 9, 2025
I found it very helpful. However the differences are not too understandable for me