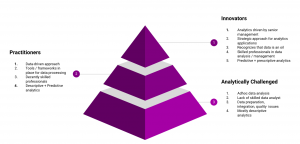
As businesses continue to embrace digital transformation, data analytics has become an essential component of business strategy. As a result, many organizations have established or are in the process of setting up an Analytics Center Of Excellence (Analytics COE) to drive analytics initiatives and improve their overall data-driven decision-making capabilities. TO achieve this objective, analytics COE requires a framework for assessing the maturity of their analytics practice. This is where analytics maturity model kicks in. The Analytics Maturity Model can be defined as a framework that enables organizations to assess their current analytics capabilities, identify areas for improvement, and establish a roadmap for analytics maturity. It provides a structured approach to understanding the key components of a successful analytics program and the stages of development that organizations typically go through.
In this post, you will learn about data analytics maturity model which you could use to assess where does your business / organization stand on the path of using analytics to drive business value. In this blog, we will dive into the Analytics Maturity Model and its three key stages of development: analytically challenged (analytics 1.0), analytics practitioners (analytics 2.0) and analytics innovators (analytics 3.0). We will explore the key characteristics and capabilities of each stage, along with the challenges and opportunities that organizations face as they progress through the model. If you represent decision-making stakeholders group and want to assess your organization readiness / capabilities to deploy analytics in order to create business value creation, you may find this post useful enough.
Here is a list of other articles I posted in the recent past in relation to strategic data analytics:
Here are the three broad categories / levels of data analytics maturity model:
- Analytically Challenged (Analytics 1.0)
- Analytics Practitioners (Analytics 2.0)
- Analytics Innovators (Analytics 3.0)
Analytics Maturity Model
Here is the diagram representing the data analytics maturity model:
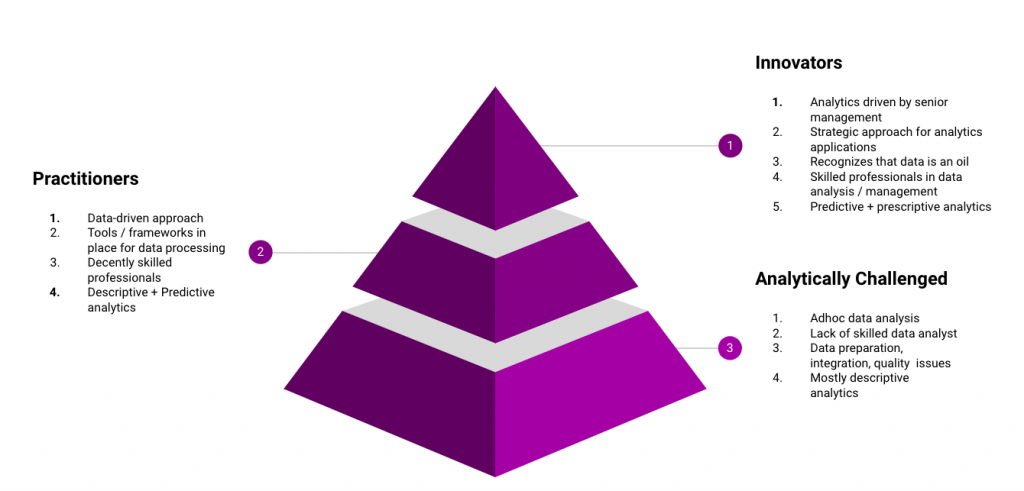
Analytically Challenged Organization (Analytics 1.0)
These are organization which are primarily using analytics for reporting purpose and also what has happened in past. When we talk about analytics approaches in terms of “look backward (what happened)” and “look forward (what might happen)”, analytically challenged organizations primarily adopt “look backward” approach. Analytics team are not part of the decision makers team. Mostly, they are part of IT organization which gets involved with creation of ad-hoc dashboards and reports based on ad-hoc demands from business teams / decision makers.
The following are some of the technologies leveraged / used by the organization at this level of analytics maturity:
- Standalone excel reports
- Data warehouse to bring in from different data sources and store data for creation of reports, OLAP cubes, etc.
- ETL tools / scripts for data processing / transformation
Here is how the analytically challenged organization would look like:
- Perform descriptive analytics: Analytics for analytically challenged organization would mostly mean descriptive analytics. Descriptive analytics is about using historical data to find patterns in the data in order to identify trends or extract actionable insights. For example, using historical sales data or spend data to identify or extract actionable insights in form of reports is descriptive analytics.
- Ad-hoc reporting & data analysis: Reports are generated in ad-hoc manner based on demand from stakeholders representing business functions. These are not created based on some sort of analytics strategy.
- Tracking business outcomes with analytics in ad-hoc manner: In the ad-hoc reporting stage, the primary analytics goal is mostly the insights which can help in tracking business outcomes in an ad-hoc / random manner.
- Data quality issues: Data quality remains one of the key issue for analytically challenged organization. Apart from data quality issue, the ability to access the data on-demand also remains as one of the key challenges.
- Lack of skilled professionals: In this stage, analytics organization does lack skilled professionals in the field of data analysis (analytical skills), usage of reporting tools, data management (sourcing / preparation / aggregation) skills etc.
Analytics Practitioners (Analytics 2.0)
As organization progresses ahead, it gets on to become practitioners and do some of the following:
- The focus moves from creating reports and dashboards to building data products covering descriptive and predictive analytics. The following includes some of the data products:
- The analytics team gets data scientists and big data experts on board.
- Business starts demanding advanced analytics (predictive analytics) solutions which are more aligned with “look forward” approach
- Big data including structured and unstructured data becomes key for analytics solutions.
- Technologies such as Hadoop, Spark becomes most sought after, for crunching big data.
The following represents some of the characteristics of organizations who could be termed as Analytics Practitioners:
- On the path of becoming data-driven organization: Ongoing initiatives to become data-driven organization; Management puts focus on laying down data analytics strategy to realize business value as a result of data analytics projects.
- Google data products such search, maps, adsense, etc
- Linkedin or Facebook data products such as people you may know
- Netflix data products such as movies you may like
- Data management & governance practices get established and practised: The analytics team work to have data management practices in place in relation to sourcing data from different internal organizations / business functions, prepare & aggregate data for extracting insights. Both structured and unstructured data starts getting processed / transformed while leveraging big data technologies such as Hadoop, Spark, etc.
- Descriptive & predictive analytics: Analytics organization starts making use of both descriptive analytics and predictive analytics to find solutions to analytics problem. Predictive analytics make use of artificial intelligence / machine learning to create models which could make predictions based on the learnings from historical data set / past experience.
- Initiatives for skilling / hiring skilled professionals including data scientists: Analytics team look out to hire skilled professionals or upskill existing employees to learn / acquire knowledge in the field of data management, descriptive analytics tools / frameworks usage, AI / ML engineers. Data scientists start getting hired at this stage of maturity as they start playing a key role.
- Operational excellence becomes key focus: Analytics focus expands its horizon to achieve operational excellence in different departments related to different business functions.
Analytics Innovators (Analytics 3.0)
The following represents some of the characteristics of organizations who could be termed as Analytics Innovators:
- Data-driven culture, data-driven products: Business stakeholders mandate it for data analytics team to work on data-based products. The key business decisions are made based on actionable insights extracted out of one or more data analytics projects. The approach to analytics from analytics innovators is found to be tied to the overall business strategy. And, this is how the analytics could provide competitive advantage to such organizations.
- ROI-based Analytics projects: Planned investment in the analytics projects with KPIs & value metrics in place to measure the ROI on analytics projects. Senior management does realise the value of data in terms of data-as-oil paradigm. Analytics team works with hypothesis-first-data-second approach for analytics projects.
- Well-established data management practices: Analytics innovators have well-established data management practices which includes some of the following:
- Get data from both internal & external sources
- Data preparation / aggregation / integration practices in place including usage of tools & frameworks
- Flexible data storage in place to facilitate ease access to data from different organizations; Presence of data lake is one of the key indicators
- Prescriptive, Predictive & Descriptive analytics: Analytics organization makes use of all form of analytics including prescriptive, predictive and descriptive analytics. Prescriptive analytics recommends actions to be taken which could impact the possible outcomes. While the predictive analytics predicts what is most likely to happen in the future, the prescriptive analytics recommends actions one could take to affect those outcomes.
- Skilled Professionals: Analytics team comprise of skilled professionals in the field of data management, AI / ML, reporting tools / frameworks, ETL methods etc.
Conclusions
Here is the summary of what you learned in relation to analytics maturity model:
- An analytics organization can be assessed based on different parameters and a maturity level can be associated.
- An analytics organization can be termed as analytically challenged, analytic practitioner and analytics innovators.
- An analytically challenged organization mostly performs ad-hoc on-demand descriptive analytics by acquiring data present with internal organizations / business function unit. The key focus at this stage is cost reduction.
- The analytical practitioners apply both descriptive and predictive analytics with the help of decently skilled professionals and data management practices to realize the business value for both, operational efficiency and cost reduction.
- The analytical innovators innovate data-based, data-driven products / solutions which help make strategic decisions. All form of analytics including prescriptive, predictive and descriptive analytics are used to create analytics solution based on hypothesis-first-data-second approach.
- Retrieval Augmented Generation (RAG) & LLM: Examples - February 15, 2025
- How to Setup MEAN App with LangChain.js - February 9, 2025
- Build AI Chatbots for SAAS Using LLMs, RAG, Multi-Agent Frameworks - February 8, 2025
I found it very helpful. However the differences are not too understandable for me