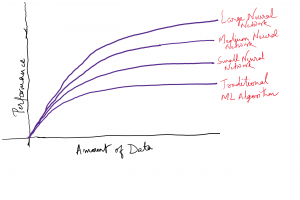
In this post, you will learn about when to go for training deep learning models from the perspective of model performance and volume of data. As a machine learning engineer or data scientist, it always bothers as to can we use deep learning models in place of traditional machine learning models trained using algorithms such as logistic regression, SVM, tree-based algorithms, etc. The objective of this post is to provide you with perspectives on when to go for traditional machine learning models vs deep learning models.
The two key criteria based on which one can decide whether to go for deep learning vs traditional machine learning models are the following:
- Model performance
- Amount of data
The following are different classes of algorithms that have been considered in this post for training the models:
- Traditional machine learning models
- Small neural networks
- Mid-size neural networks
- Large neural networks
Here is the diagram which you would want to get a good grip on when deciding between traditional machine learning vs deep learning models. This is a plot representing model performance vs the amount of data. Different curves represent different classes of models.
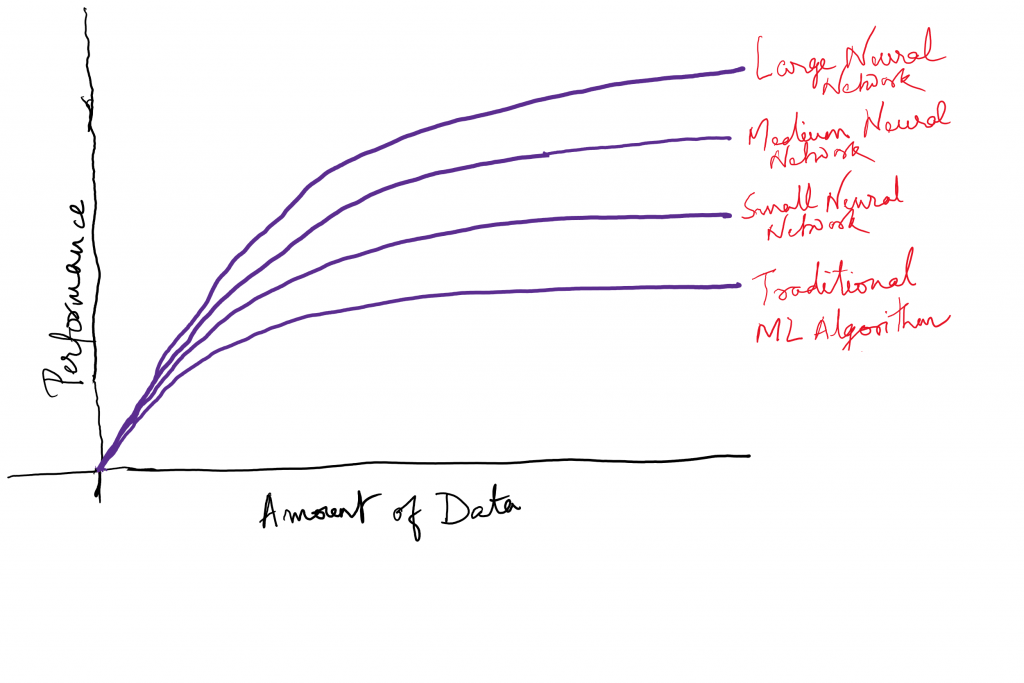
Let’s try and understand the above plot in relation to making a selection of which class of models to train.
- You may notice that the performance of all classes of model are pretty much similar if the volume of data is low.
- In case of low volume of data, one could achieve greater model performance based on some good thoughtful features while using traditional machine learning algorithms. Also, with traditional ML algorithms, one could not achieve greater model performance after a certain point irrespective of volume of data.
- Simplistic neural networks could achieve higher model performance in comparison to traditional ML algorithms if trained with huge volume of data.
- Deep (large) neural networks, however, could achieve good model performance if larger volume of data is used for training. Given that we have data coming from different sources, for complex problems, one could go for training deep neural networks to achieve high model performance.
- What is Embodied AI? Explained with Examples - May 11, 2025
- Retrieval Augmented Generation (RAG) & LLM: Examples - February 15, 2025
- How to Setup MEAN App with LangChain.js - February 9, 2025
I found it very helpful. However the differences are not too understandable for me