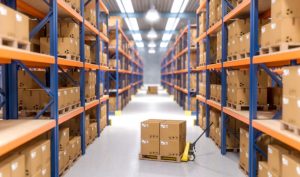
Warehouses are a vital part of the supply chain. Not only do they store products, but warehouses also play a role in shipping and receiving goods. As warehouse operations become more complex, it’s important to use technology to help manage them. Warehouses need to be able to efficiently manage the flow of goods in and out while still making room for new deliveries. Increasingly warehouses are turning to machine learning algorithms as a way to improve warehouse efficiency, reduce costs, and increase warehouse productivity. In this blog post, we will explore different machine learning use cases which can be deployed by warehouse managers to create a positive business impact.
Machine learning use cases for Warehouse management
Here are some use cases for managing warehouses in an efficient and effective manner to realize desired business impact:
- Inventory management to realize maximum savings: One of the key challenges of warehouse management is keeping track of inventory. With machine learning, warehouse managers along with the AI team can develop models to predict stock needs and plan future inventory purchases accordingly. This will help reduce the amount of excess stock that is often held in warehouses, which can lead to significant savings.
- Warehouse layout/space optimization: Warehouse managers are often challenged with optimal warehouse space planning. Machine learning algorithms and optimization techniques can be used to create predictive models which will help warehouse managers use the available warehouse space more efficiently. It is especially important for warehouses that deal in bulk items, where optimizing warehouse efficiency significantly increases the overall profitability of operations.
- Product picking optimization: Picking the right product from warehouse inventory is an important task for warehouse staff. Failing to do so will result in warehouse inefficiencies. With machine learning and optimization techniques, warehouse managers can develop algorithms that will help staff pick the correct products more quickly at the right time, and efficiently. This can lead to increased warehouse productivity and reduced costs associated with incorrect or slow product picking.
- Warehouse staff scheduling: Staff scheduling has always been an important but challenging task for warehouse managers. The problem with staff scheduling is that it is often difficult to predict warehouse workloads accurately. With machine learning algorithms and optimization techniques, warehouse managers can develop models to predict the number of staff required for different shifts and times of the day. This will help with the efficient allocation of resources and prevent any unnecessary overtime costs. This will result in increased productivity and reduced costs associated with understaffing or over-staffing.
- Predictive maintenance of warehouse equipment: Warehouses rely heavily on forklifts, warehouse carts, warehouse vehicles, and other industrial equipment to complete warehouse operations. Machine learning algorithms can be used to predict the performance of these machines based on warehouse activity data collected by connected IoT devices. This will help reduce costs associated with breakdowns or failures of warehouse equipment which causes delays in warehouse activities. Deep learning models can also be used to detect patterns in warehouse activity data which could indicate an upcoming equipment failure. This will help warehouse managers take preventive action and avoid any costly downtime of warehouse machinery.
- Routing optimization: Routing done by warehouse workers is also an area where mistakes are often made due to lack of visibility. If routing problems cause goods not to be picked up on time, warehouse managers can face penalties from suppliers. Machine learning algorithms used for warehouse management can help warehouse managers develop predictive models which will enable them to route warehouse workers more effectively and avoid those late pick-ups. Optimization algorithms such as warehouse vehicle routing or warehouse cart routing can also be developed to reduce warehouse walking distances and enhance warehouse worker productivity. This will ultimately lead to increased warehouse efficiency as well as reduced costs associated with delays in the delivery of orders/goods by customers. Multi-objective optimization can also be used to optimize warehouse worker tasks and schedules while taking into account the warehouse capacity and delivery deadlines.
- Warehouse safety: The health and safety of warehouse workers is always of paramount importance. With machine learning, warehouse managers can develop models that will help predict potential hazards in the warehouse and take preventive actions accordingly. This will go a long way in ensuring the safety of warehouse workers at all times.
Warehouse management is one of the most challenging tasks because warehouse managers have to deal with a lot of unpredictability. With machine learning, warehouse managers can predict stock needs and plan future inventory purchases accordingly. This will help reduce the amount of excess stock that is often held in warehouses, which can lead to significant savings. In this blog post you learned how machine learning algorithms for warehouse management can optimize warehouse space planning, pick products from warehouse inventory more quickly at the right time and efficiently, schedule staff more effectively so they don’t over or understaff during different days/times periods; prevent potential hazards by predicting them before they happen, and finally make routing decisions based on real-time data collected in order to avoid delays caused by picking up late deliveries. In case you want to learn more, please feel free to reach out.
- Mathematics Topics for Machine Learning Beginners - July 6, 2025
- Questions to Ask When Thinking Like a Product Leader - July 3, 2025
- Three Approaches to Creating AI Agents: Code Examples - June 27, 2025
I found it very helpful. However the differences are not too understandable for me