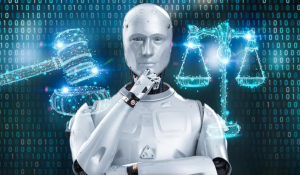
As technology continues to advance, it’s important that we prioritize ethical considerations and ensure that the development and deployment of AI technologies are responsible and fair. Meta (formerly known as Facebook) recognizes the importance of responsible AI and has taken several steps to ensure that their AI systems are developed and deployed in an ethical and fair manner.
In this blog post, we’ll be exploring the latest responsible AI updates from Meta, which every company should take into consideration when developing and implementing their own AI strategies and systems. I will keep the blog short and crisp. If you want greater details, visit this page.
Use Varied Datasets & Robust Tools to Promote Inclusivity
Organizations can learn from Meta’s dedication to creating diverse datasets and robust tools, ensuring AI solutions are inclusive, equitable, and accessible. By addressing AI biases, enhancing accuracy, and fostering inclusivity for users across various demographics, organizations can develop AI products that better serve their diverse user base and promote fairness. Here are the key actionable points:
- Diverse Datasets and Fairness: Create and distribute diverse datasets to address AI biases, improve accuracy, and ensure fairness in AI systems. By doing this, organizations can experience numerous benefits across different teams. Product teams can design solutions that cater to a broader audience, fostering customer satisfaction and loyalty. Marketing teams can leverage inclusive AI tools to create personalized and culturally sensitive campaigns, resonating with diverse customer segments. Human resources can utilize unbiased AI algorithms in recruitment and talent management processes, promoting a more diverse and inclusive workforce.
- Reliable Fairness Measurement: Develop large-scale benchmarks and methods for testing AI systems to provide a holistic view of fairness. By establishing comprehensive evaluation metrics, teams across the organization can identify potential biases and address fairness concerns more effectively. Such benchmarks and testing methods can help various teams, including research, engineering, and product development, to collaborate and make data-driven decisions, ensuring that fairness is consistently prioritized throughout the AI development lifecycle. Moreover, the insights gained from these benchmarks can inform the creation of guidelines and best practices, enabling organizations to proactively address fairness concerns and mitigate potential risks.
- Inclusive AI for Global Communication: Foster AI development to facilitate communication across languages and cultures, reflecting the diversity of end customers. By investing in AI solutions that prioritize inclusivity, companies can break down language barriers and cultural differences, enabling them to reach wider audiences and expand their global reach.
Safeguarding privacy while Tackling Fairness Issues
Organizations often face the challenge of assessing the impact of AI on demographics and addressing unfair differences while preserving user privacy. Failing to achieve this balance can result in biased AI systems and erode user trust, negatively affecting the company’s reputation and customer relationships.
Collaborating with privacy-focused companies and adopting encryption-based methods like Secure Multiparty Computation (SMPC) can help organizations find the right balance, ensuring fair AI systems without compromising user data. This approach enables secure statistical analysis while maintaining trust with customers.
Forming mindful associations
Fairness includes ensuring AI systems do not generate harmful or disrespectful content towards marginalized communities. AI-driven recommendation systems can inadvertently create harmful associations, reflecting and reinforcing biases embedded in social and semantic data.
Organization should consider creating cross-disciplinary team to understand and address problematic content associations in AI systems, reducing the chance of such occurrences on platforms using AI models. This team should review the content generated from time-to-time and constructed a searchable knowledge base of interest topics for advanced mitigations in time to come.
Allowing Users Greater Control over AI-driven Content / Recommendations
Users often feel overwhelmed by AI-driven recommendations and content, leading to dissatisfaction and disengagement. For example, users do feel overwhelmed by AI-driven recommendations and content can be found on video streaming platforms like YouTube. Users often receive an abundance of video suggestions based on their viewing history and the platform’s AI-driven recommendations. As the AI algorithm continually suggests new content, some users may find it challenging to discover content that genuinely interests them or feel bombarded by too many irrelevant or repetitive recommendations. This overwhelming experience can lead to dissatisfaction and disengagement, with users spending less time on the platform or seeking alternative sources for content.
Keeping this in mind, organizations can adopt features like Show More/Show Less and Feeds tab, allowing users to customize their experience, adjust preferences, curate favorites lists, and filter content based on their interests. This enhances user satisfaction, personalization, and engagement, creating a more enjoyable user experience.
Implement AI Explainability Methods
Here are some of the things organization can do to achieve explainability around their AI systems:
- Adopting AI Transparency Methods & Tools: Organizations can benefit from implementing tools like AI System Cards, Model Cards, and Method Cards, which offer clear explanations of AI systems, guide improvements, and ensure reproducibility for both experts and nonexperts. This approach enhances transparency and builds trust in AI systems among stakeholders. AI system cards can be defined as a concise description of an AI system’s architecture and functioning, enabling better understanding for a wide range of audiences. Model cards can be defined as up-to-date document outlining the performance, training data, and intended use of a specific AI model, promoting transparency and accountability. Method cards can be defined as a guiding document for machine learning engineers on how to address potential shortcomings, fix bugs, and improve system performance, fostering transparency and reproducibility.
- Embracing Industry Evolution: By continuously iterating on AI transparency practices, organizations can stay relevant and maintain user trust in the rapidly advancing AI landscape. Adapting to product changes and evolving industry standards demonstrates a commitment to responsible AI development and positions the organization as an industry leader.
Ensure AI Transparency with Open Loop Program
Organizations can consider implementing a similar AI governance approach to Meta’s (Facebook) global experimental governance program, Open Loop. By collaborating with clients’ companies, their competitor companies, academia, and civil society, organizations can develop and test forward-looking policies on AI transparency, explainability, and governance across various regions.
Adopting such a method offers numerous benefits to organizations. It facilitates proactive policy development, enabling organizations to stay ahead of regulatory changes and ensure compliance. Moreover, this collaboration between stakeholders promotes shared learning and best practices, fostering the development of more effective and practical AI policies.
By actively engaging with customers and partners in diverse regions, organizations can gain valuable insights into regional nuances and cultural differences, informing the development of AI systems that cater to a wider audience. In turn, this approach helps organizations demonstrate their commitment to responsible AI development and establish themselves as industry leaders in AI governance.
- Agentic Reasoning Design Patterns in AI: Examples - October 18, 2024
- LLMs for Adaptive Learning & Personalized Education - October 8, 2024
- Sparse Mixture of Experts (MoE) Models: Examples - October 6, 2024
I found it very helpful. However the differences are not too understandable for me